How do HR and Analytics get along together?
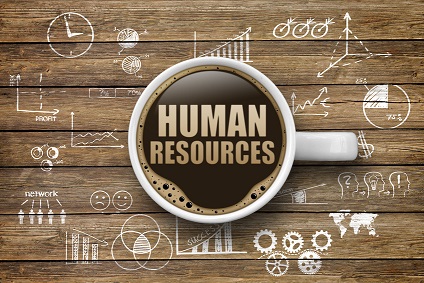
This question reminds me of a friend whose opinions I value so much. He has been trying to emphasize the importance of data and the method of using it in CRM for years. Many of his blogs mention examples of “slaughtering data” in implementations of CRM analytics. And we all know that the maturity of analytics in CRM is way above HR analytics. Unfortunately, I am quite pessimistic about the present and the future of analytics to bring correct and consistent data to HR management.
But the good thing is, whenever I talk with HR professionals, I realize that there is a consensus about the importance of analytics in HR, and “Data Driven HR” is a common concept that everybody embraces. Additionally, everyone agrees that HR management needs detailed, correct and consistent data about and around the employee in the organization to make the right decisions. Therefore, we don’t have to convince anybody about how important data is. And the size of data is ever increasing. This leads us to the inevitable fact that we need technology (moreover, integrated software) to handle, store and process relevant data consistently and sustainably.
Contributing to my pessimistic viewpoint about the current situation and the future lies behind the fact that there are too many empirical methods to choose from to select which data would be needed for what kind of HR analytic study. I would like to mention a few of my findings and conclusions as a result of many analytics studies we carried out with our clients to help organizations get the best out of their HR analytics studies, whatever their maturity level would be:
- If you are trying to do HR analytics for the sake of drawing some nice colorful charts and infographics with whatever data/information you have in hand, then please don’t. I can guarantee that your organization is going to get harmed if you do. If you don’t, at least you will know that you are deciding with your instincts and experience.
- If you think you need HR analytics, then try to identify what you want to learn and what decision(s) you are going to make using the thing(s) you learn. That is, start from the end. There is an anonymous saying that is just perfect for this: “A captain who does not know where he wants to sail, there is no wind on Earth that will bring him there.”
- After you decide about what you want to learn and how you are going to use what you learn, you will easily be able to say how you would like to measure it (that will form the parameter(s) – or another way of saying it, the critical success factors- that you will use).
- That is the point where you can identify what kind of data you are going to need specifically.
- Naturally, the parameters that you want to measure must be measurable. That is, they should have a unit, scale and reference or target quantities, amounts or percentages.
- Every parameter should be related to one or more data with some certain weights, percentages, or formulas. Or, you will be creating those formulas as a result of the analytics study.
- Although it seems like everything is easier after that, the real complication of “Analytics” starts here. Because:
- You will see that most of the time, you will come up with more than one parameter for any decision point, and more than one data to measure a parameter. Furthermore, one parameter may be relevant to more than one decision point.
- Depending on the type of your decision and many other factors, the data needed to measure these parameters will have interactions among them. Even parameters will have interactions among them. That is one result will affect the result of another.
- Moreover, the level of affects and formulas to be used will not be determined that simply. Unless you are very experienced about the subject and know the exact relation between all the data and the parameters, you are going to need time to make some trials, take measurements, and identify the relationships. That means you will need time and capability to track the changes over time.
This is where the new analytics approaches and machine learning technology start playing important roles in this process. Because, many older methods turn into simpler and faster decision-making systems and tools that enable you to get much faster results reliably and in much shorter times. I would advise you to get help from people who are really specialized in this area called “Data Scientists.” This will take you where you want to go as long as you:
- Identify clearly what you want to decide upon with what purpose.
- Determine what the parameters would be to make your decision.
- Specify your measurement methods and what kind of data you will need to gather from what kind of sources in which periods using which methods.
- Last but not the least, store the data in a consistent manner over time, so that you can cross check the results of your decisions with the resulting data collected and then duplicate the process to reach better decisions over time.
If you do not follow these basic rules, as I see happening in many organizations, you will not be able to talk about analytics in HR, but play with colorful graphics and continue to make your decisions based on your intuition, as you have always done, which is better than false number crunching anyway.